Qwen2-VL series 技术报告解读
知乎:Cassie链接:https://zhuanlan.zhihu.com/p/721908386写在前面Qwen团队在 2024年9月19日开源了Qwen2-VL-72B 模型,并发布了技术报告。这里简单介绍下“国货之光”——Qwen2-VL-72B 的技术细节。1. Contributions(1) 多语言模型Qwen 在多语言OCR任务上的表现。(2) 支持任意分辨率、比例的素材(img
知乎:Cassie
链接:https://zhuanlan.zhihu.com/p/721908386
写在前面
Qwen团队在 2024年9月19日开源了Qwen2-VL-72B 模型,并发布了技术报告。这里简单介绍下“国货之光”——Qwen2-VL-72B 的技术细节。
1. Contributions
(1) 多语言模型
Qwen 在多语言OCR任务上的表现。
(2) 支持任意分辨率、比例的素材(img + video + text)输入,在视觉评测集(DocVQA, InfoVQA, RealWorldQA, MTVQA, MathVista等)上取得SoTA结果
当前的LVLM是把输入的图片处理成固定尺寸,例如224*224,作者认为这会限制模型捕捉不同尺度信息的能力,同时会使高分辨率图像丢失太多信息;
vision encoder的训练不够,有研究通过ft vision encoder获得了模型性能的提升;
为了提升作者对不通分辨率图片的处理能力,作者移除了绝对位置编码PE,引入了2D Rotary Position Embedding。
(3) 支持img + video + text等多种模态的输入,支持20分钟以上视频输入
位置编码移除LLM中的1D-RoPE,将其分解为components: temporal, height, and width;
引入Multimodal Rotary Position Embedding (M-RoPE)。例如对text输入,distinct-IDs退化为1D-RoPE;对image输入,distinct-IDs是由height & width components决定的;对于video输入,distinct-IDs是由height & width + temporal决定的。
2. Approach
(1) 范式vision encoder(ViT) + LLM (无adapter)
模型结构。
(2) 参数量
三种规模的参数量。
3. Training details
(1) Parallelism: 同时应用Data Parallelism, Tensor Parallelism, Pipeline Parallelism, Sequence parallelism。
(2) 分布式: deepspeed zero_1。
(3) Image and Video放在一起训练。video每秒抽两帧,image每张复制一份,即image跟video保持一致一起训。vit的时候,数据用了3D convolution,从而保持帧间连贯性。
(4) 3-stage training 其中llm由QWen2初始化来的,ViT由DFN初始化来;
model | stage aim | datasets |
---|---|---|
freeze llm,train ViT | learning image-text relationships | |
unfreeze llm,train llm+ViT | facilitating a more nuanced understanding of the interplay between visual and textual information,提升关键的文字理解能力 | |
freeze ViT,train llm(instruction ft) | develop a more versatile and robust language model capable of handling complex, multimodal tasks in addition to traditional text-based interactions |
(5) datasets知识更新自202306之前。
(6) QWen2的词表新增了一些special token(e.g.,<|vision_start|>Picture1.jpg<|vision_end|>)。
4. Performance
(1) benchmark。
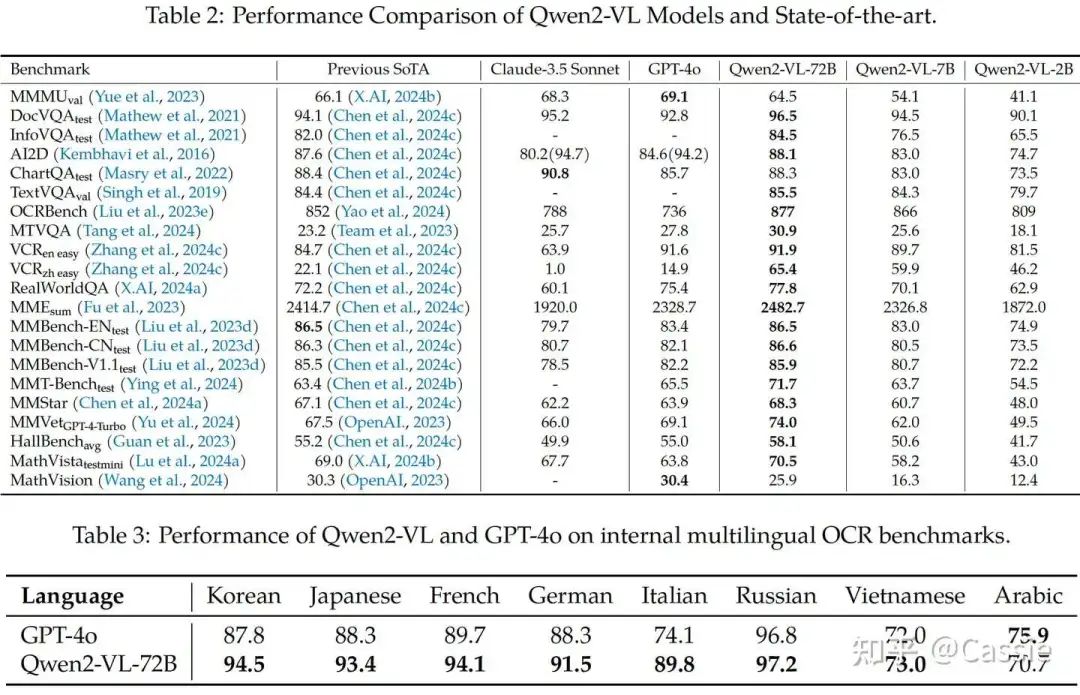
(2) 消融实验。
1D-RoPE vs M-RoPE。
model scale的表现对比。
5. Limitations
(1) 无法识别视频中的语音。
(2) 知识更新到202306。
(3) 识别知名人物和IP的能力不够。
(4) 处理复杂的多步骤任务的能力不够。
(5) 数数的能力不足。
(6) 3-D空间识别能力不足。
-1. 写在后面
(1) 资源使用。目前我在8张a100上训练Qwen2-vl-72b,用deepspeed zero_3勉强能训练起来。
不同模型规模不通训练方法的显存依赖。
参数量和显存占用大概的关系ref:大模型参数量和占的显存怎么换算?
https://www.zhihu.com/question/612046818/answer/3438795176
(2) LVLM的数据模型laws目前尚不清楚,仍然相信大力出奇迹(直观看72B明显强于7B)。
(3) 一个有意思的测评链接,结论是Pixtral擅长vision-based的问答,Qwen2-vl擅长text-based的问答。
https://ai.gopubby.com/testing-the-pixtral-model-from-mistral-67fd82810288
备注:昵称-学校/公司-方向/会议(eg.ACL),进入技术/投稿群
id:DLNLPer,记得备注呦
更多推荐
所有评论(0)