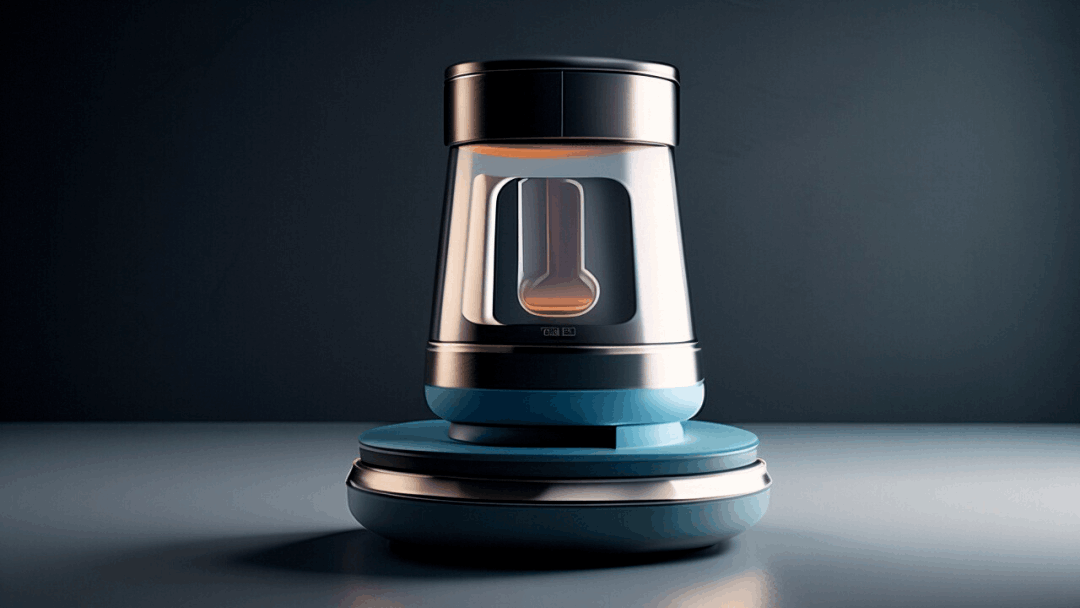
社区供稿 | GLM-4适配ModelScope-Agent最佳实践
简介 GLM-4是由智谱 AI 发布的新一代基座大模型。GLM-4 相比上一代基座模型 GLM-3 实现了 60% 的性能全面提升,直接逼近 GPT-4。一方面,GLM-4 支持更长的上下文、更强的多模态能力;另一方面,GLM-4 支持更快的推理、更多并发,大大降低了推理成本。同时,GLM-4 也增强了智能体能力,智谱 AI 正式上线了「GLM-4-All Tools」和「GLMs」个性化智能体定
简介
GLM-4是由智谱 AI 发布的新一代基座大模型。GLM-4 相比上一代基座模型 GLM-3 实现了 60% 的性能全面提升,直接逼近 GPT-4。一方面,GLM-4 支持更长的上下文、更强的多模态能力;另一方面,GLM-4 支持更快的推理、更多并发,大大降低了推理成本。同时,GLM-4 也增强了智能体能力,智谱 AI 正式上线了「GLM-4-All Tools」和「GLMs」个性化智能体定制能力,在产品上持续全面对标 OpenAI。这些全新升级后的能力,目前已经在智谱 AI 开放平台上线。(参考:最接近GPT-4的国产大模型诞生了)
ModelScope-Agent是GPTs 开源的实现方案,允许用户通过聊天、直接配置的方式进行llm的定制,可以允许用户自定义知识库以及接入web-browsing、文生图、code-interpreter等多个工具的能力。通过简化的操作,可以让更多爱好AI的人使用AI实现需求,而不需要进行具体的代码开发。ModelScope-Agent为开源社区的用户提供类似的应用构建体验,同时方便用户能够接入不同的LLM、tool,方便用户定制。(参考:魔搭开源版GPTS来啦!轻松搭建个人超级智能体!)
GLM-4接入
GLM-4接口获取
访问GLM-4官网链接获取ak和接口信息。找到我们需要的“函数调用”示例,使用ak尝试运行。
from zhipuai import ZhipuAI
client = ZhipuAI(api_key="") # 请填写您自己的APIKey
tools = [
{
"type": "function",
"function": {
"name": "query_train_info",
"description": "根据用户提供的信息,查询对应的车次",
"parameters": {
"type": "object",
"properties": {
"departure": {
"type": "string",
"description": "出发城市或车站",
},
"destination": {
"type": "string",
"description": "目的地城市或车站",
},
"date": {
"type": "string",
"description": "要查询的车次日期",
},
},
"required": ["departure", "destination", "date"],
},
}
}
]
messages = [
{
"role": "user",
"content": "你能帮我查询2024年1月1日从北京南站到上海的火车票吗?"
}
]
response = client.chat.completions.create(
model="glm-4", # 填写需要调用的模型名称
messages=messages,
tools=tools,
tool_choice="auto",
)
print(response.choices[0].message)
得到如下返回结果
content=None role='assistant' tool_calls=[CompletionMessageToolCall(id='call_8323270002625790606', function=Function(arguments='{"date":"2024-01-01","departure":"北京南站","destination":"上海"}', name='query_train_info'), type='function')]
接入ModelScope-Agent
(具体代码可查看modelscope_agent/llm/zhipu.py文件)
- 新建ZhipuLLM 继承BaseChatModel类。在ZhipuLLM类中需要实现如下函数:
- __init__函数:在__init__中可将zhipuai通用信息初始化,如ak(api_key)的获取和检查、ZhipuAI客户端的初始化。
- chat函数(包括_chat_stream和_chat_no_stream):由于同机构不同模型的接口基本一致,在ZhipuLLM类中需要实现zhipuai特有的_chat_stream和_chat_no_stream分别进行ZhipuAI下大模型的流式和非流式调用。
- chat_with_functions函数(非每个模型必须):如果function_call是通过文本信息传入,前两点的实现已经能够支持一个模型运行。由于ZhipuAI 函数调用的接口形式为function_call单独传入,需要额外实现chat_with_functions函数,供function作为参数传入的处理。
import os
from typing import Dict, Iterator, List, Optional
from zhipuai import ZhipuAI
from .base import BaseChatModel, register_llm
@register_llm('zhipu')
class ZhipuLLM(BaseChatModel):
"""
Universal LLM model interface on zhipu
"""
def __init__(self, model: str, model_server: str, **kwargs):
super().__init__(model, model_server)
self._support_fn_call = True
api_key = kwargs.get('api_key', os.getenv('ZHIPU_API_KEY', '')).strip()
assert api_key, 'ZHIPU_API_KEY is required.'
self.client = ZhipuAI(api_key=api_key)
def _chat_stream(self,
messages: List[Dict],
functions: Optional[List[Dict]] = None,
tool_choice='auto',
**kwargs) -> Iterator[str]:
if not functions or not len(functions):
tool_choice = 'none'
print(f'====> stream messages: {messages}')
response = self.client.chat.completions.create(
model=self.model,
messages=messages,
tools=functions,
tool_choice=tool_choice,
stream=True,
)
return stream_output(response, **kwargs)
def _chat_no_stream(self,
messages: List[Dict],
functions: Optional[List[Dict]] = None,
tool_choice='auto',
**kwargs) -> str:
if not functions or not len(functions):
tool_choice = 'none'
response = self.client.chat.completions.create(
model=self.model,
messages=messages,
tools=functions,
tool_choice=tool_choice,
)
return response.choices[0].message
def chat_with_functions(self,
messages: List[Dict],
functions: Optional[List[Dict]] = None,
stream: bool = True,
**kwargs) -> Dict:
functions = [{
'type': 'function',
'function': item
} for item in functions]
if stream:
return self._chat_stream(messages, functions, **kwargs)
else:
return self._chat_no_stream(messages, functions, **kwargs)
在实现上述第二点 流式访问时,我们需要对流式输出的返回进行处理。处理内容主要为:将调用的function_call的api名和参数、或返回的文本内容,组织成我们想要的格式。此外,状态检查、fallback_call等拓展后续也方便在此处展开。
def stream_output(response, **kwargs):
func_call = {
'name': None,
'arguments': '',
}
for chunk in response:
delta = chunk.choices[0].delta
if delta.tool_calls:
# TODO : multi tool_calls
tool_call = delta.tool_calls[0]
print(f'tool_call: {tool_call}')
func_call['name'] = tool_call.function.name
func_call['arguments'] += tool_call.function.arguments
if chunk.choices[0].finish_reason == 'tool_calls':
yield {'function_call': func_call}
else:
yield delta.content
考虑到每个模型可能有其特殊处理,GLM4 继承自ZhipuLLM,可重写其方法。此处GLM4无特殊处理,仅注册@register_llm('glm-4')
@register_llm('glm-4')
class GLM4(ZhipuLLM):
"""
glm-4 from zhipu
"""
为使modelscope-agent框架找到新注册的ZhipuLLM和GLM4 两个类,需要在modelscope_agent/llm/__init__.py 增加对新文件的import。
此时,GLM-4已接入modelscope-agent。
Agentfabric调试
Agentfabric核心使用了roleplay(modelscope_agent/agents/role_play.py)和AgentBuilder(modelscope_agent/agents/agent_builder.py)两个agent。其中前者用于用户自定义agent,用户可通过入参赋予agent的角色身份、特点描述、功能/流程等;后者用于构建一个rolepaly agent,用户通过与AgentBuilder对话,让AgentBuilder生成roleplay所需的角色身份、特点描述、功能/流程等描述。
因此,可通过在这两个agent上的测试,看看glm-4适配效果
Roleplay
在此同时测试工具调用,这里的示例工具为天气查询,需要申请高德天气api-key 并配置到环境变量AMAP_TOKEN中
# tests/tools/test_weather.py
from modelscope_agent.agents.role_play import RolePlay
def test_weather_role():
role_template = '你扮演一个天气预报助手,你需要查询相应地区的天气。'
# model和model_server名称需严格与类定义时的注册一致(如 @register_llm('glm-4')、@register_llm('zhipu'))
llm_config = {'model': 'glm-4', 'model_server': 'zhipu'}
# input tool name
function_list = ['amap_weather']
bot = RolePlay(
function_list=function_list, llm=llm_config, instruction=role_template)
response = bot.run('朝阳区天气怎样?')
text = ''
for chunk in response:
text += chunk
print(text)
assert isinstance(text, str)
test_weather_role()
Answer:Action: amap_weather
Action Input: {"location":"朝阳区"}
Observation: <result>朝阳区的天气是晴温度是0度。</result>
Answer:朝阳区的天气是晴温度是0度。
Agent_builder
需要模型根据用户需求对json进行填空,对输出格式有严格要求。这里主要测试结构化生成能力。
# tests/agents/test_agent_builder.py
from modelscope_agent.agents import AgentBuilder
def test_agent_builder():
# model和model_server名称需严格与类定义时的注册一致(如 @register_llm('glm-4')、@register_llm('zhipu'))
llm_config = {'model': 'glm-4', 'model_server': 'zhipu'}
# input tool name
function_list = ['image_gen']
bot = AgentBuilder(function_list=function_list, llm=llm_config)
response = bot.run('创建一个多啦A梦')
text = ''
for chunk in response:
text += chunk
print(text)
assert isinstance(text, str)
assert 'Answer:' in text
assert 'Config:' in text
assert 'RichConfig:' in text
test_agent_builder()
Answer: 您希望这个多啦A梦AI-Agent具备哪些特殊的能力和功能?例如,它的口袋里应该有哪些神奇的道具?它应该在哪些场景下帮助用户?请具体说明。
Config: {"name": "", "description": "", "instructions": [], "prompt_recommend": [], "logo_prompt": ""}
RichConfig: {"name": "多啦A梦AI-Agent", "description": "一个基于著名动漫角色的AI-Agent,拥有各种神奇的道具和能力,旨在帮助用户解决问题,带来欢乐。", "instructions": ["理解用户的请求并提供相应的帮助", "根据用户需求使用不同神奇道具", "在学习和生活中为用户出谋划策"], "prompt_recommend": ["多啦A梦,我需要你的帮忙!", "你能用任意门带我去旅行吗?", "我想要一个记忆面包,帮助我记住所有知识点", "今天天气不好,能用晴天娃娃给我带来阳光吗?"], "logo_prompt": "一个圆头圆脑,穿着蓝色机器猫服装的角色,胸口有一个四维口袋,背景是充满科技感的环形轨道"}
效果展示
链接访问:
https://modelscope.cn/studios/modelscope/AgentFabric/summary
或 在apps/agentfabric 运行app.py
(本地运行需要自己配置zhipu、天气工具和画图工具的api-key)
选择模型glm-4,配置指令“你扮演一个天气预报助手,你需要查询相应地区的天气,并调用给你的画图工具绘制一张当前天气下城市的图。”,点击“更新配置后”,在右侧预览对话框输入“朝阳区天气怎样?”。
点击直达Agent创建体验:Agent创建专用 · 创空间 (modelscope.cn)
更多推荐
所有评论(0)